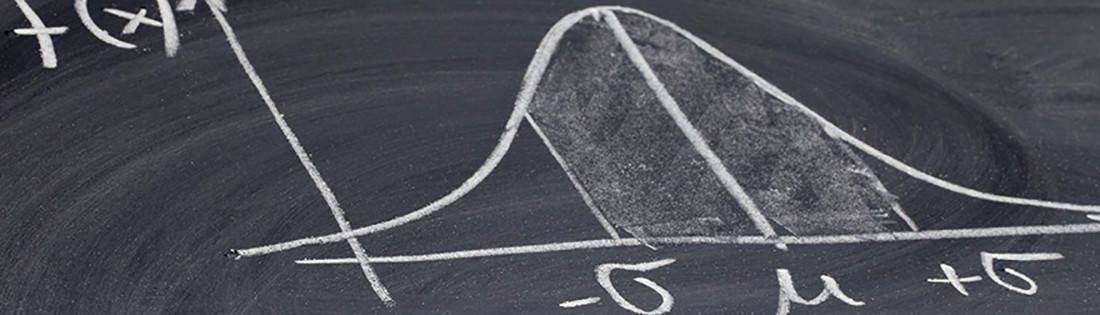
Stochastic Systems
This area of research is concerned with systems that involve uncertainty. Unlike deterministic systems, a stochastic system does not always generate the same output for a given input. Stochastic systems are represented by stochastic processes that arise in many contexts (e.g., stock prices, patient flows in hospitals, warehouse inventory/stocking processes, and many others).
This area includes:
Queueing Systems: Analysis and design of service systems with uncertainty in the arrival of “customers,” which could include people, materials, or information, and the system’s capacity to process arrivals given the uncertainty in processing time.
Markov Decision Processes: Dynamic and sequential decision making processes that take actions in real-time to achieve optimal performance under conditions of uncertainty and ambiguity about the future.
Reliability and Maintainability: Analysis of systems that are failure-prone and design of systems to achieve high reliability with minimum resources under conditions of risk.
RELATED NEWS
-
Berahas Receives ONR Grant to Develop Advanced Optimization Algorithms
The grant will fund the development of cutting-edge algorithms for solving complex nonlinear constrained stochastic optimization problems. Albert Berahas, and Micheal O’Neill will lead the research to advance optimization methods and address real-world challenges.
-
Do Third-Party Delivery Services Benefit Restaurants?
U-M IOE researchers discovered that a restaurant can benefit from partnering with third-party delivery services if at least one of five conditions applies to them.
-
Kati Moug receives 2021 Generation Google Scholarship
U-M IOE PhD student, Kati Moug, has received a 2021 Generation Google Scholarship in recognition of academic performance, leadership, and a commitment to diversity, equity and inclusion.
-
Viswanath Nagarajan receives NSF funding to research a new class of stochastic optimization problems
U-M IOE Assistant Professor Viswanath Nagarajan has received NSF funding to explore models and algorithms for stochastic covering problems in the presence of noisy outcomes.
-
How to hack your deadline: Admit it’s uncertain
Two simple steps can take the fear out of drop-dead dates.