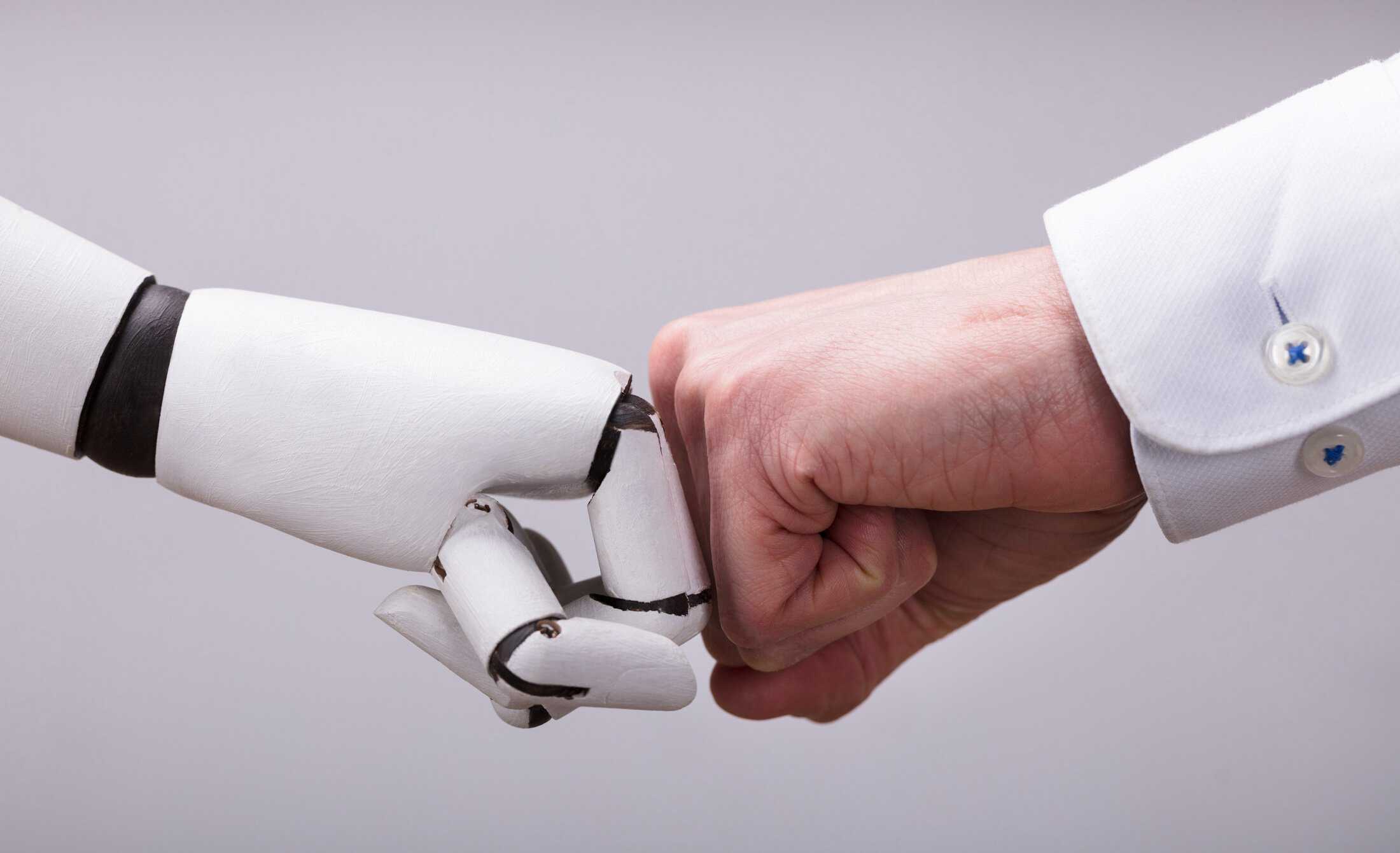
Building trust between humans and robots when managing conflicting objectives
When robots do not have prior knowledge of human tactics, adaptive learning enhances trust and performance.
IOE-STAGE SITE
When robots do not have prior knowledge of human tactics, adaptive learning enhances trust and performance.
A new University of Michigan study on how humans and robots work together on tasks with conflicting objectives is the first to demonstrate that trust and team performance improve when the robot actively adapts to the human’s strategy.
Conflicting objectives involve trade-offs such as speed vs. accuracy. Aligning to the human’s strategy was most effective for building trust when the robot did not have prior knowledge of the human’s preferences.
The study was presented today at the Human-Robot Interaction Conference in Boulder, Colorado.
The algorithm the researchers developed can extend to any human-robot interaction scenario involving conflicting objectives. For instance, a rehabilitation robot must balance a patient’s pain tolerance with long-term health goals when assigning the appropriate level of exercise.
“When navigating conflicting objectives, everybody has a different approach to achieve goals,” said Xi Jessie Yang, an associate professor of industrial and operations engineering and last author on the paper.
Some patients may want to recover quickly, increasing intensity at the cost of higher pain levels, while others want to minimize pain at the cost of a slower recovery time.
If the robot doesn’t know the patient’s preference for recovery strategy ahead of time, using this algorithm, the robot can learn and adjust exercise recommendations to balance those two goals.
This research is part of a larger body of work aiming to shift robots from a simple tool for an isolated task to a collaborative partner by building trust.
Previous research has focused on designing robots to exhibit trustworthy behaviors, such as explaining their reasoning for an action. Recently, the focus shifted to aligning robot goals to human goals, but researchers have not tested how goal alignment impacts outcomes.
“Our study is the first attempt to examine whether value alignment, or an agent’s preference for achieving conflicting objectives, between humans and robots can benefit trust and human-robot team performance,” said Yang.
To test this, study participants were asked to complete a video-game-like scenario where a human-robot team must manage conflicting objectives of finishing a search mission as quickly as possible while maintaining a soldier’s health level.
The participant assumes the character of a soldier moving through a conflict area. An aerial robot assesses the danger level within a building, then recommends whether the human should deploy a shield robot when entering. Using the shield maintains a high health level at the cost of taking extra time to deploy.
The participant accepts or rejects the robot’s recommendation, then provides feedback about their trust level of the recommendation system ranging from zero to complete trust.
The experimenters tested three robot interaction strategies:
They performed two experiments, one where the robot had well-informed prior information about the human’s strategy preferences and one where it started from scratch.
Robot adaptive learning enhanced the human-robot team when the robot started from scratch, but not when the robot had prior information, leaving little room to improve upon its strategy.
“The benefits manifest in many dimensions, including higher trust in and reliance on the robot, reduced workload and higher perceived performance,” said Shreyas Bhat, a doctoral student of industrial and operations engineering and first author of the paper.
In this scenario, the preferences of the human do not change over time. However, strategy may shift based on the circumstances. If there’s very little time remaining, a shift to increase risk-taking behavior can save time to help complete the mission.
“As a next step, we want to remove the assumption from the algorithm that preferences stay the same,” said Bhat.
As robots become more integral in conflicting objective tasks in fields like healthcare, manufacturing, national security, education and home assistance, continuing to assess and improve trust will strengthen human-robot partnerships.
Additional co-authors: Joseph B. Lyons of the Air Force Research Laboratory and Cong Shi of the Miami Herbert Business School.
This research was funded by the Air Force Office for Scientific Research.Full citation: “Evaluating the impact of personalized value alignment in human-robot interaction: Insights into trust and team performance outcomes,” Shreyas Bhat, Joseph B. Lyons, Cong Shi, X. Jessie Yang, ACM/IEEE International Conference on Human Robot Interaction (2024). DOI: 10.48550/arXiv.2311.16051